Project 1
Quantum annealing based optimization for machine learning
This project focuses on benchmarking D-Wave quantum annealers for particular use cases in optimization and machine learning. The benchmarking approach is three-part: different generations of D-Wave quantum annealers that are accessible via JUNIQ, the quantum computer user facility at FZJ, are compared with each other, and the results are compared with results obtained on conventional computers and with results obtained using a hybrid approach, i.e. the combined use of a quantum annealer and a conventional computer.
The hybrid approach is used to define use cases for the modular supercomputing architecture and thus connect WP1 with WP2. Additional cross-platform benchmarking uses gate-based quantum computing to solve reduced versions of the optimization and machine learning problems solved by the D-Wave quantum annealers.
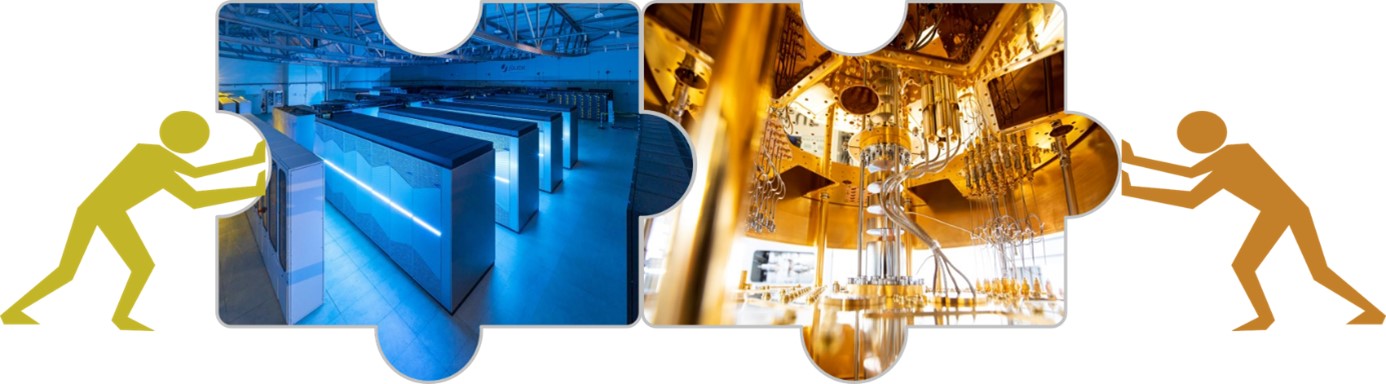
Integration of high performance computing and quantum computing for practical quantum computing with hybrid quantum-classical algorithms.
Studied use cases include:
- Solving the maximum matching problem for scheduling
- Remote sensing image classification by quantum support vector machines
- Feature data transformation
Team for Quantum Computing
Prof. Dr. Kristel Michielsen
WP leader
Prof. Dr. Kristel Michielsen
k.michielsen@fz-juelich.de
FZJ, JSC
Dr. Stéphane Louise
WP deputy
Dr. Stéphane Louise
Prof. Dr. Gabriele Cavallaro
research personnel
Prof. Dr. Gabriele Cavallaro
g.cavallaro@fz-juelich.de
FZJ, JSC
Dr. Renaud Sirdey
research personnel
Dr. Renaud Sirdey
Dr. Madita Willsch
postdoctoral researcher
Dr. Madita Willsch
m.willsch@fz-juelich.de
FZJ, JSC
Valentin Gilbert
PhD student
Valentin Gilbert
Berat Yenilen
master student
Berat Yenilen
b.yenilen@fz-juelich.de
FZJ, JSC
Prof. Dr. Morris Riedel
research personnel
Prof. Dr. Morris Riedel
m.riedel@fz-juelich.de
FZJ, JSC
Edoardo Pasetto
PhD student
Edoardo Pasetto
e.pasetto@fz-juelich.de
FZJ, JSC
Links to websites:
https://www.fz-juelich.de/en/ias/jsc/about-us/structure/research-groups/qip